Embracing Disruption
Cloud computing is essential to fulfilling generative AI’s potential
Generative AI (Gen-AI) is a game-changing technology having an “iPhone moment,” thanks to its potential to disrupt and transform entire industries. But generative AI needs the kind of data and processing power that only cloud computing can provide, which can create attractive investment opportunities.
HIGHLIGHTS
- The great promise of generative AI requires immense amounts of data and significant computational power — which only cloud computing hyperscalers may be able to handle. Many other sub-industries (including semiconductors, semiconductor equipment and software) will likely all be beneficiaries of this new ecosystem.
- This is an “iPhone moment,” with generative AI poised to positively impact not only hardware, software and security, but also how consumers create and absorb information.
- AI-enabled industries could be key beneficiaries of generative AI growth catalysts across cloud-based software and services.
Generative AI (Gen-AI) is a game-changing technology having an “iPhone moment,” thanks to its potential to disrupt and transform entire industries. But generative AI needs the kind of data and processing power that only cloud computing can provide, which can create attractive investment opportunities.
Why cloud computing is central to AI’s future
The transformative power of artificial intelligence (AI) and the already perceptible profound developments and changes, it is presently triggering in various areas of life and work will require significant computational power and infrastructure, not only to train AI models but to help companies make use of them.
AI is an umbrella term that covers a variety of technologies and training techniques (natural language processing (NLP), virtual agents, robotic process automation, deep learning, machine learning, etc.).
And they all need immense amounts of data and significant computational power to train and run their models. Only cloud hyperscalers — large-scale cloud service providers — can handle the enormous data workloads. (To train the GPT-4 model, the hardware alone was estimated to cost USD 100 million.1) But even these massive firms will need to expand as data volume and complexity continues to grow.
Gen-AI technologies, primarily large language models, are no exception. The machine learning model of which ChatGPT is run was trained on 45 terabytes (TB) of text data, which is the equivalent of 1 million feet of bookshelf space. The developers used approximately 175 billion model parameters (configuration variables). As the parameter count increases with model development, the output accuracy rate increases and it expands the business customization possibilities significantly (Exhibit 1). The amount of data that Gen-AI models will work with is only expected to increase. Consider that about 2.5 quintillion bytes (10^18) of data are created every day globally — an amount that is expected to double every two to three years.2 The true power of Gen-AI lies in its ability to convert this data into a useful tool for companies and consumers.
Exhibit 1: Each GPT iteration uses more trainable parameters, which increases accuracy and expands used cases
GPT trainable parameters
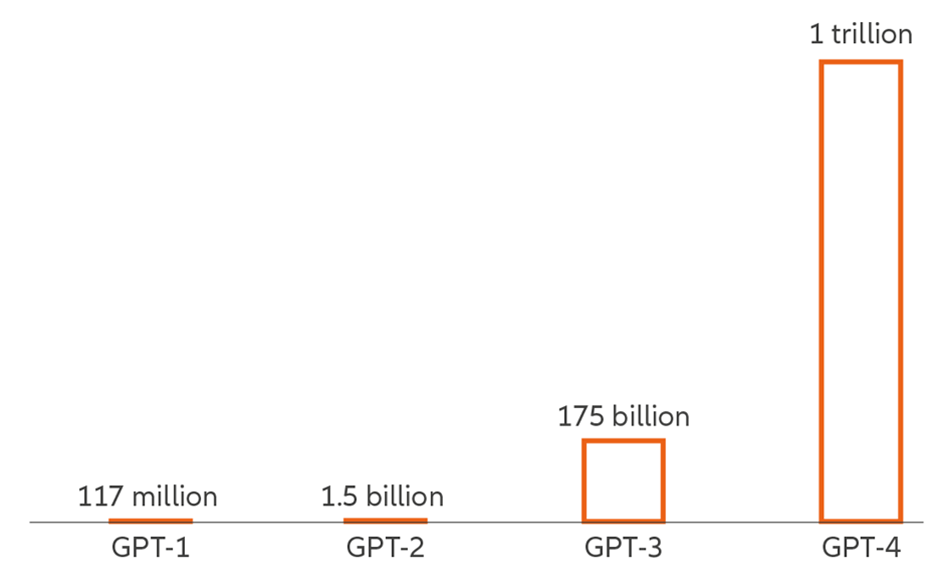
Source: OpenAI. GPT= Generative pre-trained transformer, as of March 2023.
While it’s true that public cloud spending has slowed as of late — given macro-economic pressures, the industry’s mid-cycle growth rates and a rising rate environment — the portion related to AI is expected to increase (Exhibit 2).
- The total volume of cloud spending related to AI is expected to grow substantially over the medium term to over USD 300 billion by 2026.
- Of the total addressable market of AI related spending, the portion dedicated to cloud infrastructure and services is expected to grow from 50% today to almost 60%, reflecting the data intensive nature of future models and inference uses.
- RBC Capital Markets projects an increase of 26% in the overall cloud providers total addressable market due to generative AI.3
Quite simply, as generative AI grows, nearly all the required data will need to funnel through the hyperscaler providers. However, given the power of the technology and its applicability across business and consumer use, a new cadre of native Gen-AI applications will need to be developed to address the sheer number of possible used cases, both at present and ones that have not even been thought of yet.
Why AI and cloud computing matter for investors
Although cloud spending was forecast to continue double digit growth for the foreseeable future, most investors agreed that the industry had entered its mid stage lifecycle. Despite GPT specific models being developed and trained since 2017/18, it wasn’t until the release of the ChatGPT and image applications such as DALL-E and Midjourney that the near-term potential of generative AI “shocked” both consumers and company management into understanding how transformative and disruptive this technology will most likely be.
As a result, there is a rapid assessment taking place of which companies have been preparing for these moments for some time, which ones are new to Gen-AI, but have the ability to still take advantage, and those companies which are either late to the game or fail to see the benefits. That’s why some industry watchers are saying the recent advances in Gen-AI are an “iPhone moment”. With its 2007 introduction, Apple’s flagship product introduced a new era of hardware and software development on the tech side, but also dramatically changed consumption patterns and multi-generational consumer preferences.
Exhibit 2: Overall public cloud spending is projected to rise steadily…
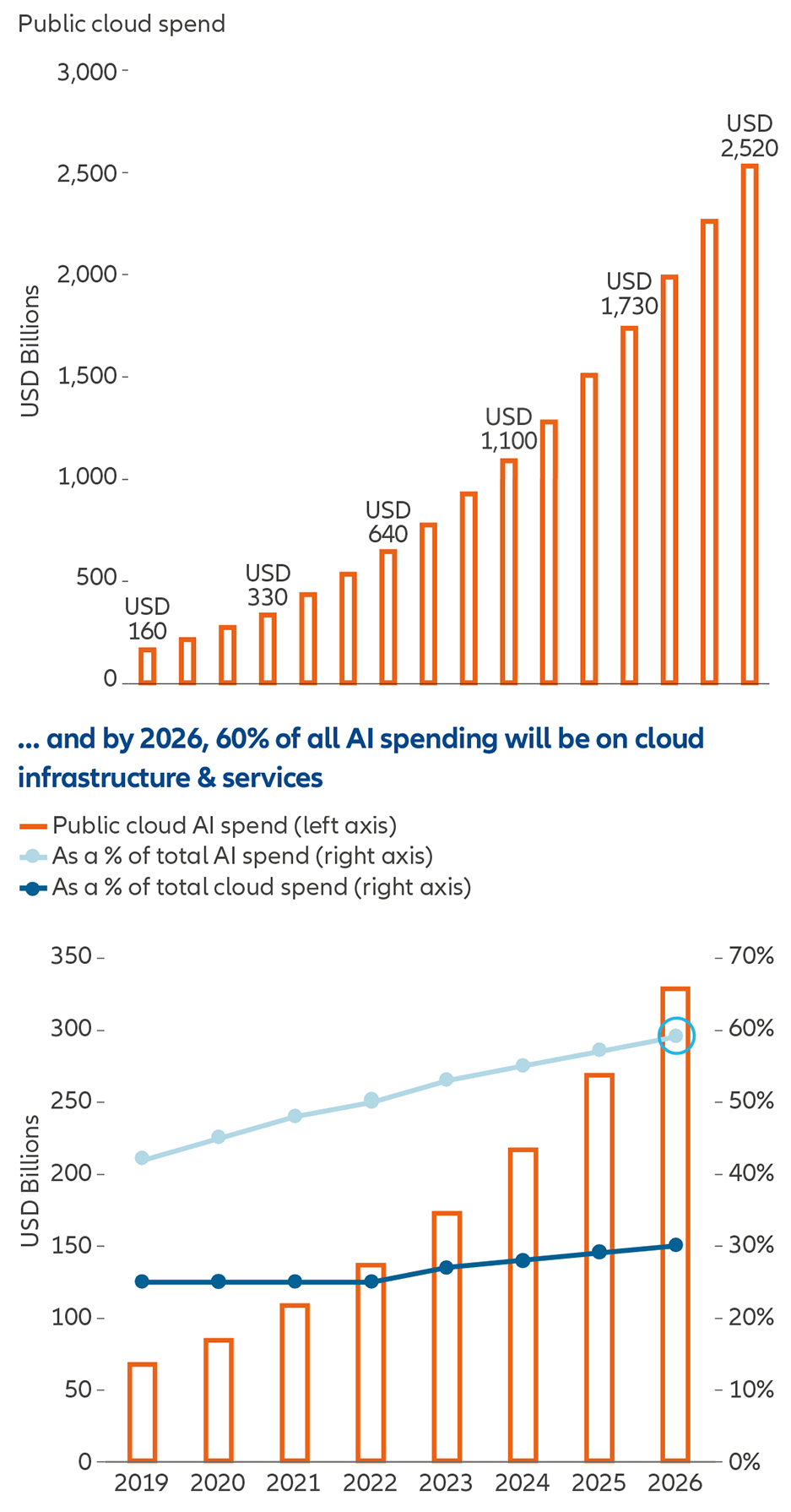
Source: Morgan Stanley Research, IDC, as of March 2023.
As AI experiences its “iPhone moment,” we believe Gen-AI will not only positively impact technology hardware (advanced GPUs, data centers, Edge Computing), software (cloud service providers, SaaS, customization), and security (data protection, data audits, regulatory compliance), but also alter how consumers create and absorb audio, visual, and textual information. In addition, Gen-AI should have a unique impact (relative to the iPhone) on how businesses utilize the technology to increase productivity and streamline workflows. After cloud services became mainstream in 2006 with the advent of Amazon Web Services, we believe generative AI could be the next major catalyst for growth in cloud computing.
How Allianz Global Artificial Intelligence is positioned
Although iterations of the technology of generative AI have existed for decades, specific applications such as ChatGPT and DALL-E represent important milestones, especially in the area of unsupervised machine learning and deep learning applications.
The advancements around generative AI have proliferated at a pace that far exceeds prior tech cycles we have experienced in our nearly 30 years of technology investing. We believe we are at an inflection point where corporations will accelerate their deployment of AI to change their business models.
In the last months, the deployment of practical AI tools such as GPT plugins and chatbots has quickened, as have the discussions around implications for businesses, employees and investors. Through the middle of May, there have been nearly 1,600 mentions of “AI” by US and European firms during their first quarter earnings calls — a record by a significant margin. In May, Goldman Sachs issued a report4 estimating a 400 basis-point improvement in aggregate profit margins for US companies over the next decade due to the incorporation of various AI technologies, but especially generative AI.4
Exhibit 3: In earnings calls in 1Q23, a huge spike in mentions of “AI” and “cloud”
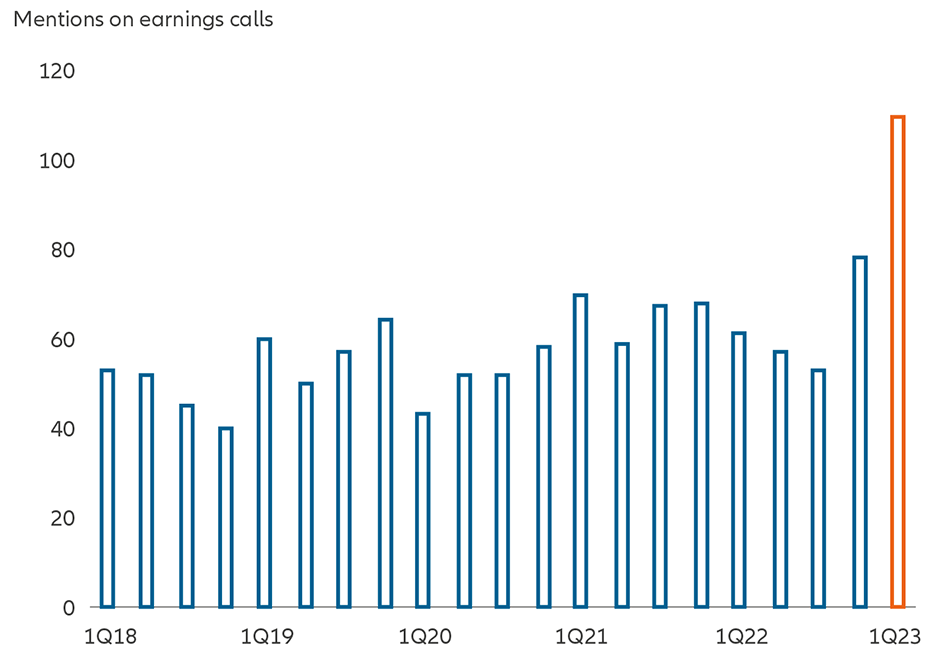
Source: Bloomberg, as of March 2023..
Based on a recent Grassroots Research® survey of 300 IT decision makers5, AI was voted as the technology that will have the greatest impact on their business over the next five years. In addition, 49% said their company uses generative AI for work purposes and 29% are looking for ways to do so. Among respondents who have used it, 56% use it for analyzing data and information, 52% for customer support, and 48% for brainstorming and developing new ideas. In terms of near-term spending priorities, cloud computing was the 2nd highest. The results of this report bolster our contention that generative AI is at an inflection point and that the greatest near-term investment opportunities are in the AI infrastructure and AI applications categories.
AI-enabled industries could be key beneficiaries of generative AI as a growth catalyst positioned
As generative AI is fundamentally changing the technology landscape at an unprecedented pace, semiconductor and component makers should see benefits as the computational demands from the large language models underlying generative AI systems are orders of magnitude larger than existing infrastructure is capable of supporting. Meanwhile, many software companies have released generative AI modules helping customers automate previously rote tasks and generate valuable new insights from their data.
Over the longer term, we believe AI-enabled industries to be key beneficiaries as they leverage large language models to gain efficiencies in their operations and focus their revenue generating efforts on the highest yielding areas. Given the expansive and transformative power of generative AI, especially in the near and medium term, utilizing multiple categories should hopefully give investors a more diversified and comprehensive approach to the opportunity set.
What exactly is generative AI?
Generative AI refers to a form of machine learning (ML) called “deep learning.” This form of AI encompasses machines that are trained on sets of data to perform certain tasks and/or make predictions without human direction. An important technological advancement is the shift from supervised to unsupervised learning.
Most initial ML models involved supervised learning, in which humans were needed to classify, or “tag,” data — for example, identifying an image as a “dog” or a social media post as “political.” To be sure, this type of ML still occurs, but recent developments in generative AI are examples of unsupervised learning in which the model makes its own predictions based on massive amounts of training data.
Using the example above, the machine has moved from being able to identify the dog in an image to creating an image of the dog. Other examples of this are Dall-E creating paintings based on descriptive words using only its vast trove of image data as well as ChatGPT writing economic commentary or even computer code. Ironically, the increasing size and complexity of data sets enhance the use cases of ML (as we are seeing in ChatGPT and similar applications) in business operations and development.
1 Me, Myself and AI — Artificial Intelligence Primer, Bank of America, February 2023.
2 Bank of America, February 2023.
3 Software and Internet Applications of Generative AI and Chat GPT, RBC Capital Markets, March 16, 2023.
4 Bloomberg, 17 May 2023.
5 Grassroots Research “Global IT Spending Survey,” May 2023. Based on a survey of 300 IT professionals at companies with 100 or more employees. The GRASSROOTS RESEARCH® division of Allianz Global Investors commissions investigative market research for asset management professionals. Research data used to generate GRASSROOTS RESEARCH® reports are received from independent, third-party contractors who supply research that, as far as permissible by applicable laws and regulations, may be paid for by commissions generated by trades executed on behalf of clients